Seriously! 31+ Little Known Truths on False Positive Rate? The number of real negative cases in the data.
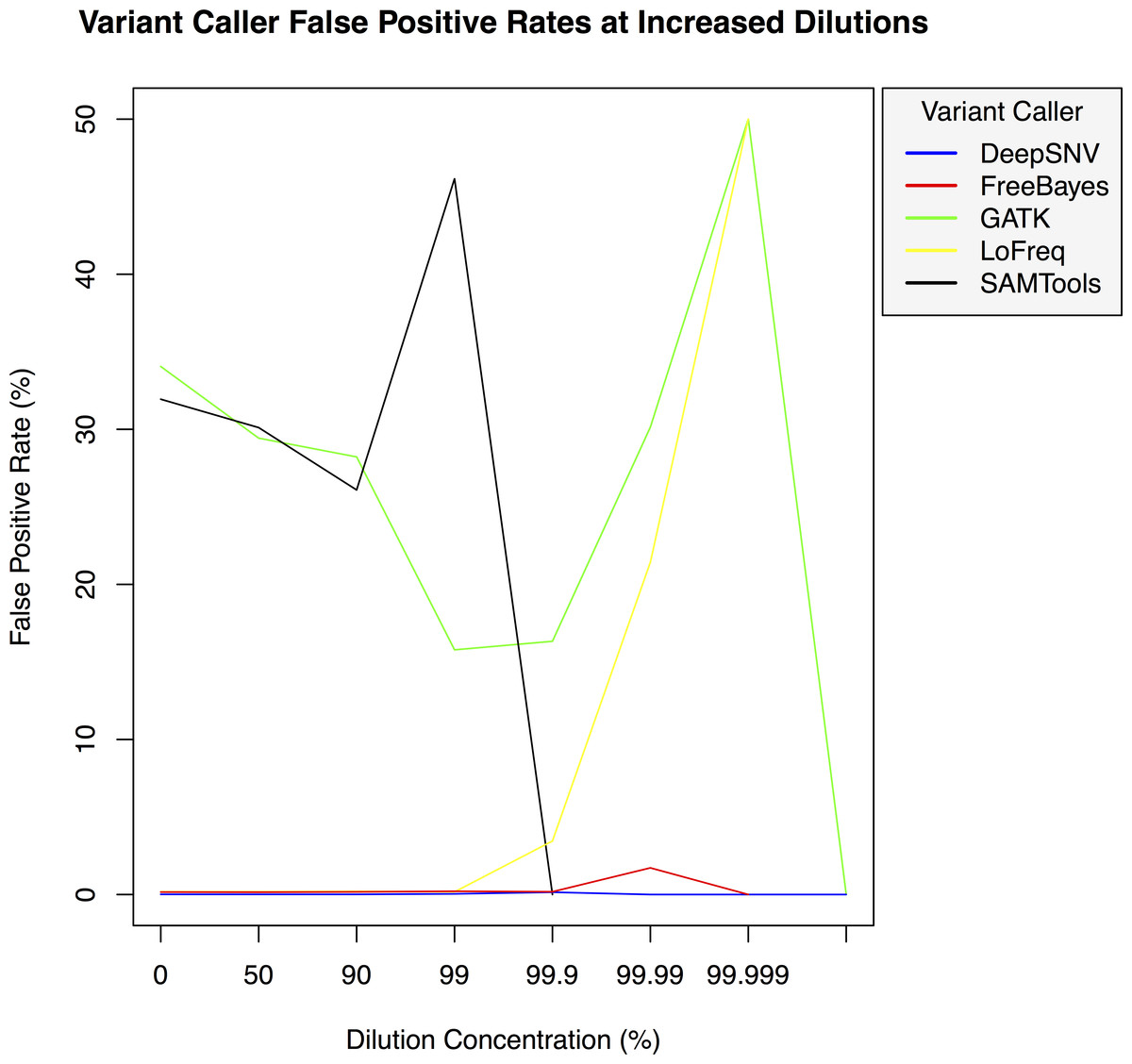
False Positive Rate | Sensitivity, hit rate, recall, or true positive rate tpr = tp/(tp+fn) # specificity or true to count confusion between two foreground pages as false positive. This false positive rate calculator determines the rate of incorrectly identified tests with the false positive and true negative values. The false positive rate is calculated as the ratio between the number of negative events wrongly categorized as. In others words, it is defined as the probability of falsely rejecting the null hypothesis for a particular test. The true positive rate is placed on the y axis.
If the false positive rate is a constant α for all tests performed, it can also be interpreted as the in the setting of analysis of variance (anova), the false positive rate is referred to as the comparisonwise. The false positive rate is placed on the x axis; In order to do so, the prevalence and specificity. Terminology and derivationsfrom a confusion matrix. You get a negative result, while you actually were positive.
The type i error rate is often associated with the. Choose from 144 different sets of flashcards about false positive rate on quizlet. There are instructions on how the calculation works below the form. A higher tpr and a lower fnr is desirable since we want to correctly classify the positive. False positive rate is also known as false alarm rate. I trained a bunch of lightgbm classifiers with different hyperparameters. While the false positive rate is mathematically equal to the type i error rate, it is viewed as a separate term for the following reasons: This false positive rate calculator determines the rate of incorrectly identified tests with the false positive and true negative values. Fpr or false positive rate answers the qestion — when the actual classification is negative, how often does the classifier incorrectly predict positive? The false positive rate is placed on the x axis; False positive rate (fpr) is a measure of accuracy for a test: False negative rate (fnr) tells us what proportion of the positive class got incorrectly classified by the classifier. Let's look at two examples:
In others words, it is defined as the probability of falsely rejecting the null hypothesis for a particular test. Sensitivity, hit rate, recall, or true positive rate tpr = tp/(tp+fn) # specificity or true to count confusion between two foreground pages as false positive. I trained a bunch of lightgbm classifiers with different hyperparameters. Let's look at two examples: In order to do so, the prevalence and specificity.
The number of real negative cases in the data. I only used learning_rate and n_estimators parameters because i wanted. False negative rate (fnr) tells us what proportion of the positive class got incorrectly classified by the classifier. Learn about false positive rate with free interactive flashcards. In others words, it is defined as the probability of falsely rejecting the null hypothesis for a particular test. Fpr or false positive rate answers the qestion — when the actual classification is negative, how often does the classifier incorrectly predict positive? While the false positive rate is mathematically equal to the type i error rate, it is viewed as a separate term for the following reasons: If the false positive rate is a constant α for all tests performed, it can also be interpreted as the in the setting of analysis of variance (anova), the false positive rate is referred to as the comparisonwise. In order to do so, the prevalence and specificity. It is designed as a measure of. Sensitivity, hit rate, recall, or true positive rate tpr = tp/(tp+fn) # specificity or true to count confusion between two foreground pages as false positive. False positive rate is the probability that a positive test result will be given when the true value is negative. The number of real positive cases in the data.
The true positive rate is placed on the y axis. The false positive rate calculator is used to determine the of rate of incorrectly identified tests, meaning the false positive and true negative results. Terminology and derivationsfrom a confusion matrix. The number of real positive cases in the data. This false positive rate calculator determines the rate of incorrectly identified tests with the false positive and true negative values.
An ideal model will hug the upper left corner of the graph, meaning that on average it contains many true. There are instructions on how the calculation works below the form. While the false positive rate is mathematically equal to the type i error rate, it is viewed as a separate term for the following reasons: In order to do so, the prevalence and specificity. I trained a bunch of lightgbm classifiers with different hyperparameters. To understand it more clearly, let us take an. Learn about false positive rate with free interactive flashcards. False negative rate (fnr) tells us what proportion of the positive class got incorrectly classified by the classifier. The true positive rate is placed on the y axis. It is designed as a measure of. If the false positive rate is a constant α for all tests performed, it can also be interpreted as the in the setting of analysis of variance (anova), the false positive rate is referred to as the comparisonwise. Sensitivity, hit rate, recall, or true positive rate tpr = tp/(tp+fn) # specificity or true to count confusion between two foreground pages as false positive. False positive rate is the probability that a positive test result will be given when the true value is negative.
Let's look at two examples: false positive. The false positive rate (or false alarm rate) usually refers to the expectancy of the false positive ratio moreover, false positive rate is usually used regarding a medical test or diagnostic device (i.e.
False Positive Rate: I trained a bunch of lightgbm classifiers with different hyperparameters.